
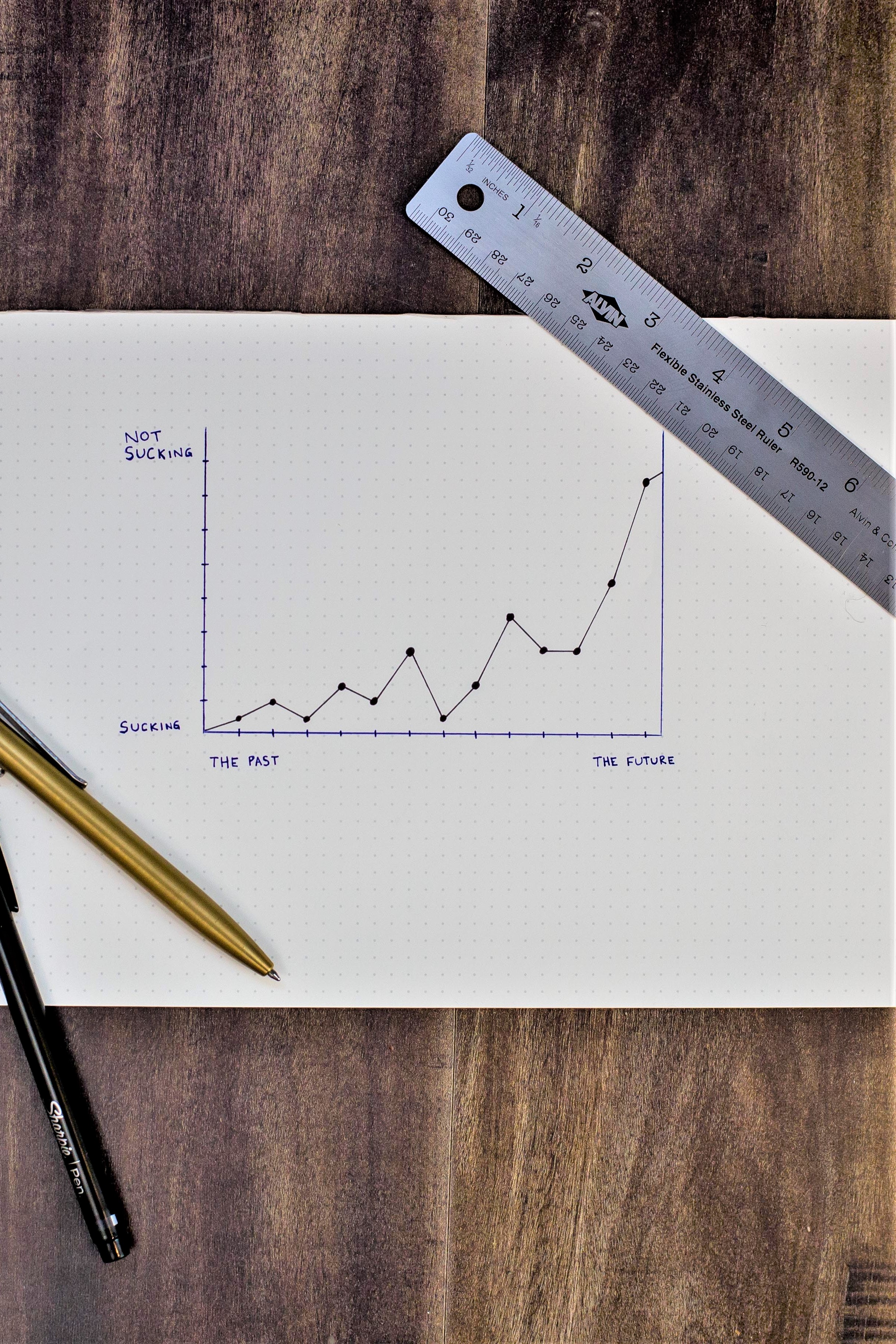
The advanced visualizations require programming. The charts of Excel can meet basic graphics requirements, but this is only the basis. There is a saying in data analysis: the text is not as good as the table, and the table is not as good as the graphic. Data visualization is one of the main directions of data analysis. And the visualization package under development, ggplot, mimics the very famous ggplot2 in R. In fact, Python mimics many features of R, such as DataFrame of Pandas. If you can solve problems efficiently with R, then use R. Maybe you hear in some places that Python is more commonly used at work, but solving problems is the most important. But I still recommend that you take a look at both. If you can only choose one of them to learn because of the limited time, I recommend using Python.
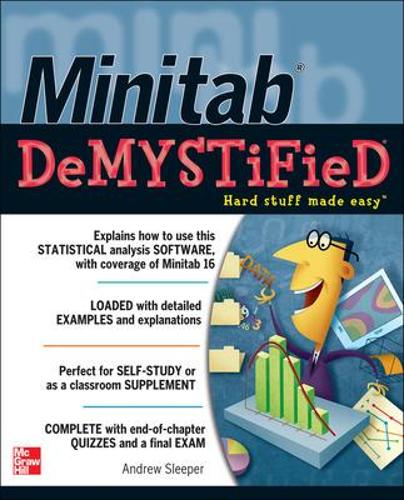
Wait until you master one of them, and then start learning another one. Wait until one of them has mastered a certain degree and then start learning another one. So it is recommended not to learn them meanwhile. But if you learn both at the same time, it will be very confusing because they are very similar in many places. So, if you are still newbie and haven’t started learning yet, I suggest you start with Python.īoth Python and R are easy to learn. The development of Python in data analysis has modeled some of the features of R in many places. But R still maintains an advantage in the field of statistics. In some respects, it has surpassed R, such as machine learning, text mining and other areas of programming. Python can also be used to develop web pages, develop games, develop system backends, and do some operation and maintenance work.Ī current trend is that Python is catching up with R in the field of data analysis. The difference is that R is used exclusively in the field of data analysis, while scientific computing and data analysis are just an application branch of Python. R and Python are both data analysis tools that need to be programmed. Advanced fields of data mining and analysis, such as machine learning and text mining.Construct data analysis algorithms based on the business scenarios and actual problems.By reading R’s help files and the information on the network, you can solve specific problems relatively quickly. With these bases, when you encounter actual problems, you can find the R package you need to use. 10 days of centralized learning is enough for mastering the basic use, basic data structure, data import and export, and simple data visualization. Data analysis report output (R markdown)įrom my point of view, getting started with R is very simple.Statistical modeling (linear regression, logistic regression, tree model, neural network, etc.).Statistical hypothesis testing (t test, analysis of variance, chi-square test, etc.).As far as our general data analysis or academic data analysis work is concerned, the things that R can do mainly include the following aspects. The functions of R cover almost any area where data is needed. Unlike Python, R and other open source softwares, there is a charge for the genuine Excel.The built-in statistical analysis is too simple and has little practical value.The Excel data file itself can hold only 1.08 million rows without the aid of other tools, and it’s not suitable for processing large-scale data sets.When the amount of data is large, there will be a situation of stuttering.

To fully master Excel, you need to learn VBA, so the difficulty is still very high.It can help you understand the meaning of many operations before further learning other tools (such as Python and R).You can do a lot of things with Excel: modeling, visualization, reports, dynamic charts, etc.Production of charts for some business magazines and newspapers (data visualization).Combine Word and PowerPoint to create data analysis reports.Simple statistical analysis for students or teachers (such as analysis of variance, regression analysis, etc.).Data management and storage of small and medium-sized companies.
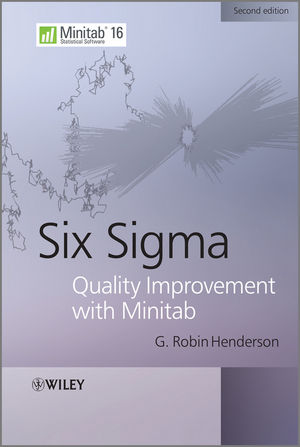
